How to Build Your Recommendation Engine
For as long as there has been commerce, vendors have been considering how to present their merchandise to potential customers. In the physical world, this was referred to as ‘merchandising,’ and the only potential personalization available was by actual intervention from a shop assistant.
Audience segmentation in advertising allowed further thought about how different content would be presented in the different media channels depending upon the preferred audience. However, in the digital world, it is possible that merchandising, and indeed all of the digital content, can be tailored for each individual. The process to do this is, of course, data.
There are degrees to how far a recommendation engine can and should go. Each level of sophistication invariably leads to a higher cost (both to develop and maintain), and so, building the business case is key.
Admittedly, Netflix has the world’s most famous (and expensive) recommendation engine — but they value its output at over $1 billion per year, which makes for a solid ROI. But not every business has the luxury of that level of return.
One thing to remember is that outside of the recommended ROI, there is a secondary and highly beneficial aspect that should be factored into those business cases: the intelligence that the company gains about its market and how that information can be monetized.

Recommendation Engine Pathway
Here are a few steps that you should consider before you proceed down the path of a recommendation engine:
1. Business Case
The first step should be to consider the business case. The revenue aspect of the business case should examine the following:
Customer Recommendations
How much will your revenue change due to better customer recommendations?
Are you anticipating selling more products to your current clients, or do you envision those recommendations may attract new customers?
Your ultimate goal should be:
- Reducing minutes of discovery for customers who aren’t sure what they want
- Exposure of the customer to an entire category that they would never have considered on their own
- Swiftly moving a new customer from ‘consideration’ to ‘purchase,’ and then later from ‘purchase to ‘re-engagement.’
Customer Intel
Product Intel
Obviously, if the platform collects data on the customer, then the same is true of the products, as well. However, it is worth noting that the product data can be much richer and even more powerful than the customer data.
Now, let’s talk about the many versions of a recommendation engine, and keep in mind that they usually entail several models that run in parallel.
The first model may be a content-based collaborative filtering model.
What makes this model interesting is that it can return affinity values of a product feature, for a given user, or group of users. Then, that can be used to predict the success of other products, or even be used to design a new product or service — based on the menu of product features. In any case, how the recommendation engine will be judged for success needs to be documented and defined. Note that the recommendation engine is not in itself responsible for the revenue increase.
The recommendation engine only provides new information to a person. Changing their behavior is what drives value, and changing behavior is a lot more complicated than just presenting new information.
2. Data Gathering
As said, there are likely to be several models of various degrees of complexity working in parallel. Each of these models will require different data.
A possible (and non-exclusive) example of this would be the ability to tell if the customer is enjoying the content they’ve viewed.
It is often assumed that users will provide their attitude to the content that they are experiencing by filling out a rating system. Most users, particularly regular users, sometimes find this to be cumbersome and tend to engage disproportionately when they have an extreme rating to give (zero or five – and more often when it is negative). This data can still be useful but requires more complex algorithms to understand and correct for them. The following affinity may be more cost-effective:
Linger
Measuring the average duration that a person spends on a page, and taking note when the ‘linger’ time exceeds the mean by one standard deviation (or two) is very useful. Just be aware of a user who ‘lingers’ for four or more standard deviations; they may have walked away to have lunch.
Return Visit
This one is self-explanatory. When someone returns to a page, they’re most likely seriously considering a purchase.
‘Buy’ or Enquire
Now, back to actions that the user might make on the page, actually buying the product, or enquiring for further information (including reading reviews) is a strong indicator of intent.
Content Attributes
How do you describe a product? Putting this into context, you may imagine the Netflix recommendation engine as only being able to describe the content as a TV show or a film. If this was the case, Netflix may inappropriately recommend a horror movie after watching Casablanca simply because they’re both films.
This doesn’t happen because Netflix looks at many details of Casablanca in order to make the recommendation. Attributes analyzed would include the medium (film), color (black or white film), actors (lead actors), director, year, genre, and many other attributes. The same logic applies to all content, products, and services. The more meaningful detail of the product you can devise, the more powerful your recommendation engine will be.
Once all of the above have been considered and documented, you’re ready to start designing your recommendation engine strategy that weighs up the data you have available with the upside of what you want it to achieve and the downside of the cost of development.
DMI’s Recommendations
1. Start with an MVP
Don’t build an overly complex feature-rich prototype to work out the math. Just get a simple model into full production to learn how it works and impacts user behavior. Then, consider additional features.
2. Rules-Based
A rules-based model can be extremely effective in the correct circumstances for a very modest investment. This will allow you to observe whether recommendations change behavior.
See ‘other considerations: quality of content’.
3. Content Filtering
One of the first steps is to bring in a model that uses the content you are looking at and to make recommendations of similar content based on the menu of attributes. This is relatively inexpensive and can be effective, but it can also lead to ‘echo chambers’ where a user gets trapped in a particular category or style of content.
4. Collaborative Filtering
It is much more interesting to use the results of other models to make recommendations that go beyond what the given user has done themselves.
5. Content-Based Collaborative Filtering
This model will build a matrix of the user’s affinity to attributes and make recommendations of content with attributes made up of those that the user has shown the most affinity towards.
6. User-Based Collaborative Filtering
In this model, a user’s appreciation for content (or categories or attributes) is compared to other users. Where there are gaps in a given users matrix, those gaps are predicted based on what similar users have done. In this way, a user may discover content categories that they would have never observed themselves.
7. Future Iterations
All of the above can start life as a simple MVP version with complexity developed over time as the organization learns about its effectiveness.
Other Considerations: Integration With Your Overall Data Strategy
There is a quirk in recommendation engines. If all of your content is amazing, you do not need a recommendation engine. Everyone will love everything they engage with. Likewise, if all of your content is terrible, the best recommendation engine in the world won’t help you. People will only dislike what you recommend to them slightly less than what they were looking at. So, if your engine doesn’t change behavior, look beyond the data scientist to find the reason why.
By leveraging automation and real-time machine learning, DMI can help you boost revenue and customer loyalty through an end-to-end recommendation engine that fits your needs. From developing the necessary data architecture to designing the front-end customer experience, our team has deep expertise in the design, development, testing, and deployment of recommendation systems. Contact us to learn more.
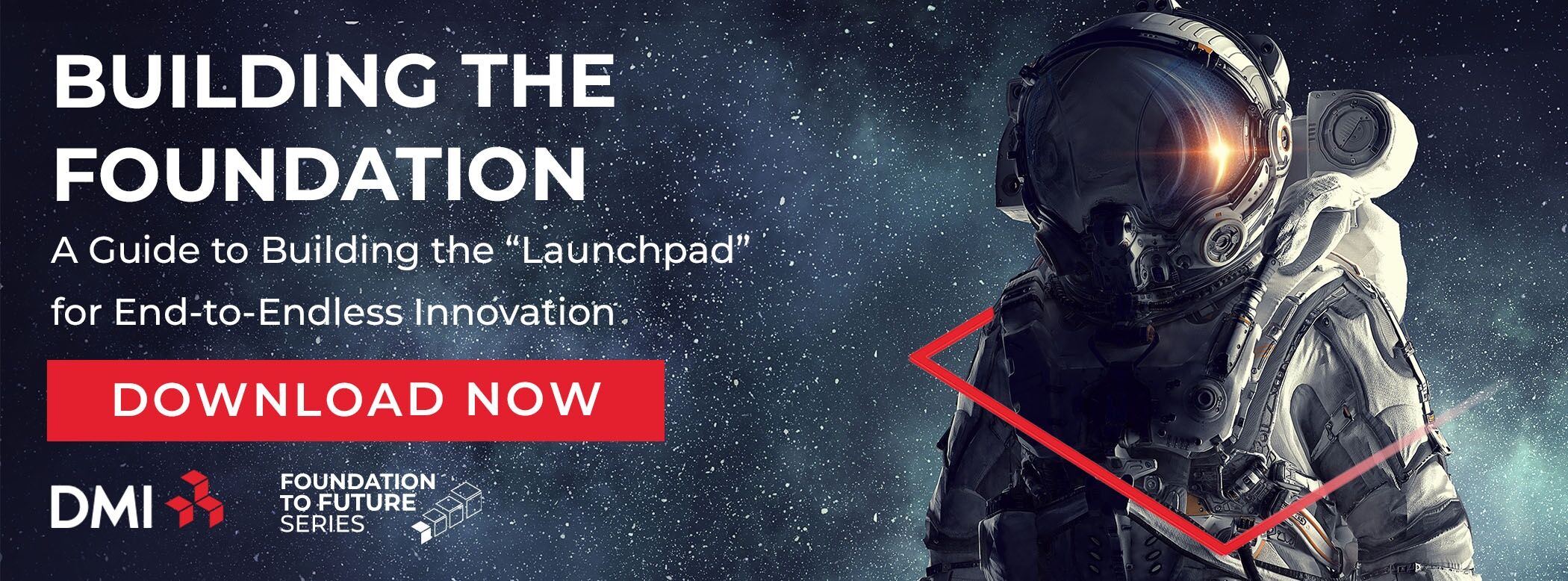